‘AI for Good’ shouldn’t become the new innovation arbitrage
If technology is being tested more aggressively in poorer communities, it's worth asking why.
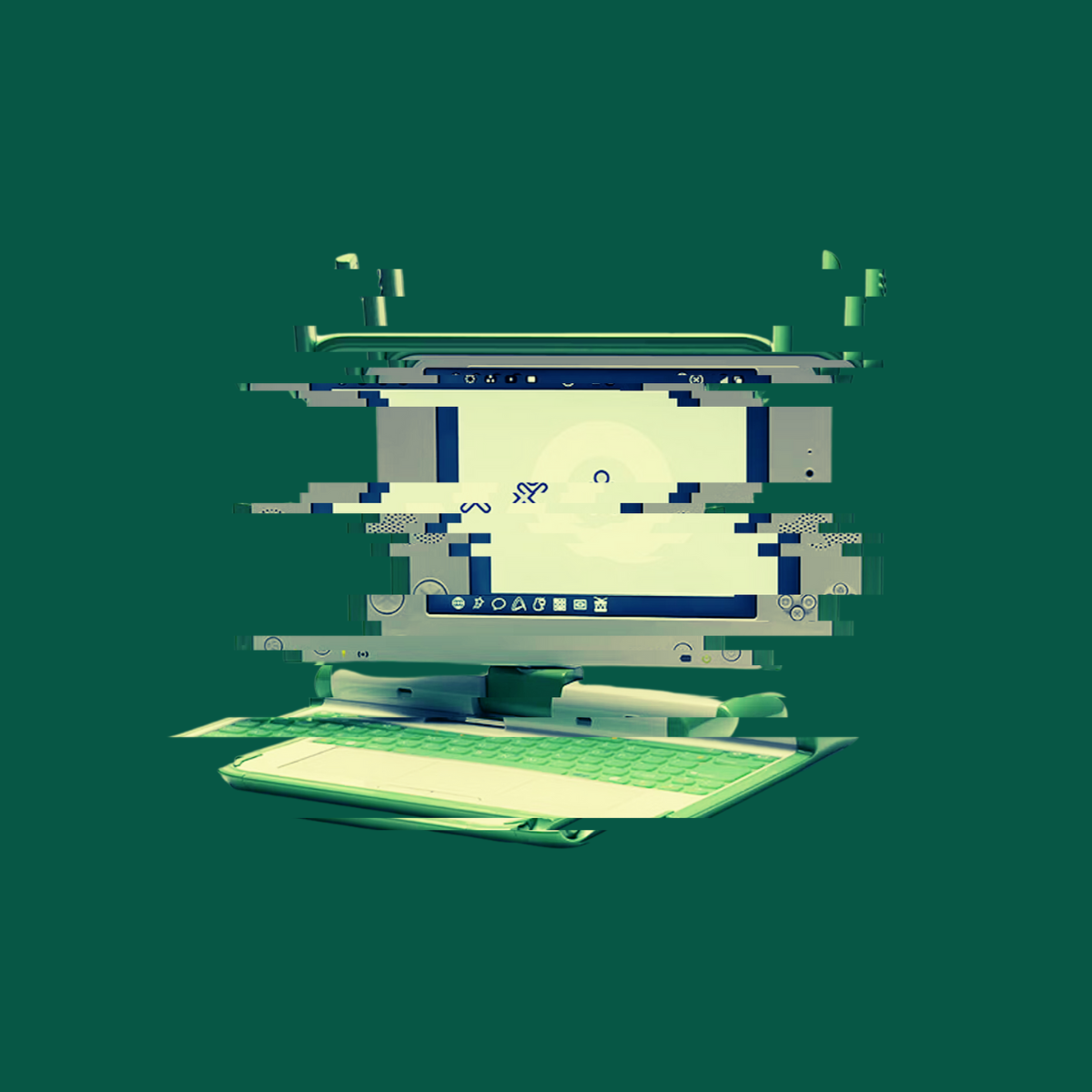
COMMENTARY By Rumman Chowdhury
At the AI Action Summit in Paris last week, tech leaders and civil society alike shared stages to tout the benefits that artificial intelligence can bring to the Global Majority. When asked for specifics on how AI will improve humanity, CEOs and tech leaders overwhelmingly point to improvements in industries like health care and education in low-resource regions. However, without careful attention to how this tech is deployed, we run the risk of using the Global Majority as a testing ground for incomplete AI solutions that exacerbate disparities in access and quality of care in these industries and others.
It’s understandable to see why AI in education and health care may be appealing. It is difficult to find qualified individuals willing and able to live and work in impoverished regions, and locals who do receive medical or educational training often seek more economically secure and upwardly mobile employment prospects elsewhere. Those that stay often end up being overworked. Inevitably, a teacher handling a classroom of 50 children may not have the resources to work with a child with a learning disability. Medical professionals may not be equipped to diagnose complex medical issues with more obscure symptoms.
AI is well poised to close these gaps. We have seen prototypes of customized learning AI tutors providing unlimited educational content. We have seen telemedicine enable rural doctors to perform surgeries and improve patient care. However, if these technologies are predominantly used in lower-income neighborhoods but applied more carefully in affluent ones, it warrants extra scrutiny. Deployed poorly, so-called “AI for Good”—or the deployment of automated systems meant to have a social impact—can serve as a convenient test environment for these tools in a less visible or legally restrictive environment, with local populations as collateral damage.
The term “innovation arbitrage” refers to the careful exploitation of regulatory gaps to deploy questionable technologies. More resourced economies may have stricter regulations around labor, privacy or data management. It becomes easier to test novel technologies in less developed economies with the added benefit that it’s unlikely that egregious failures in rural regions will be picked up by major media the way they would for a more affluent population. Uber is a perfect example. The company had an explicit strategy to test manipulative algorithms to improve driver productivity in countries where worker protection laws were weakest, while paying as little as possible.
Innovation arbitrage allows companies to gather data to improve their algorithms with little to no consequence, and enables them to refine their product for more affluent markets, where data protection laws, liability laws and more can prevent them from at-scale testing. Without the appropriate safeguards, AI for Good initiatives can be easily manipulated to serve as a new method of innovation arbitrage.
The problem is twofold. First, our definition of “digital public infrastructure” focuses on “capacity building”—that is, data collection and curation, computational resources and access to models. We rarely discuss tools for testing and evaluation to determine if these products are safe, secure and responsibly deployed.
AI for Good initiatives offer large prizes of computational resources and funding for technology teams who are passionate about the problem they’re working on and are embedded within their communities. However, these teams often skip hiring individuals who are best able to test for security, privacy and ethical flaws. It is assumed that technology teams will fix such issues post-deployment if they deem them critical enough. This means that whether these mistakes are prioritized is not driven by any legal or ethical imperatives to protect consumers, but by staffing capacity and funding. At the same time, the companies that produce these foundational models are able to gather hard-to-acquire test data from these communities.
Second, generative AI algorithms introduce a host of possible failures, far beyond the limited algorithms deployed by Uber and others in earlier iterations of innovation arbitrage. These AI tools can confidently reflect embedded biases or completely hallucinate responses. Without adequate quality checks or clearly defined methods of testing, developers risk solving the most visible problems instead of the most impactful ones.
My nonprofit, Humane Intelligence, has conducted tests to examine biases within AI tools with a wide range of communities, and has identified significant issues that can arise when well-intended technologies are launched with inadequate testing and evaluation. Testing methods for Generative AI models are poorly defined, with no clear standards or thresholds for harm identification or mitigation. The few tests that exist are largely Western-focused, for example, identifying “bias” by American racial constructs while ignoring forms of non-Western bias like caste. These limitations have real-world implications.
Along with Singapore’s Infocomm Media Development Authority, we examined a range of large language models that demonstrated significant regional biases in an Asian context. These biases arise because the individuals training and testing the core models on which educational bots are built simply lack local cultural context. Imagine an AI tutor deployed in rural parts of Malaysia that confidently states that individuals from the primarily rural eastern regions are less hygienic and more likely to make poor economic decisions because of their cultural shortcomings.
Utilizing AI as a tool of equity requires more than determining if a problem is answerable at scale. Funding data, computational resources and model development are necessary starting points, but equitable care does not mean simply providing access where there was none before. Any concept of equity requires an assessment of quality. Expecting the impoverished to be grateful for any handout, delivered enthusiastically but executed poorly, is a reflection of the savior complex that often pervades tech-for-good communities. In addition, enabling unfettered AI deployment opens the door to a global testbed of subjects to be manipulated at will, in a revived form of innovation arbitrage. This is not to say AI cannot be used for good, but that AI for Good investments also need to fund context-specific evaluation, safety and security measures.
Dr. Rumman Chowdhury is CEO and co-founder of Humane Intelligence, and the first person to be appointed by the Department of State as the United States Science Envoy for Artificial Intelligence.