We need to rethink trade secrecy to build better AI
Trade secrecy isn’t just about keeping AI models under wraps—it actively encourages secrecy, stifles competition and limits innovation.
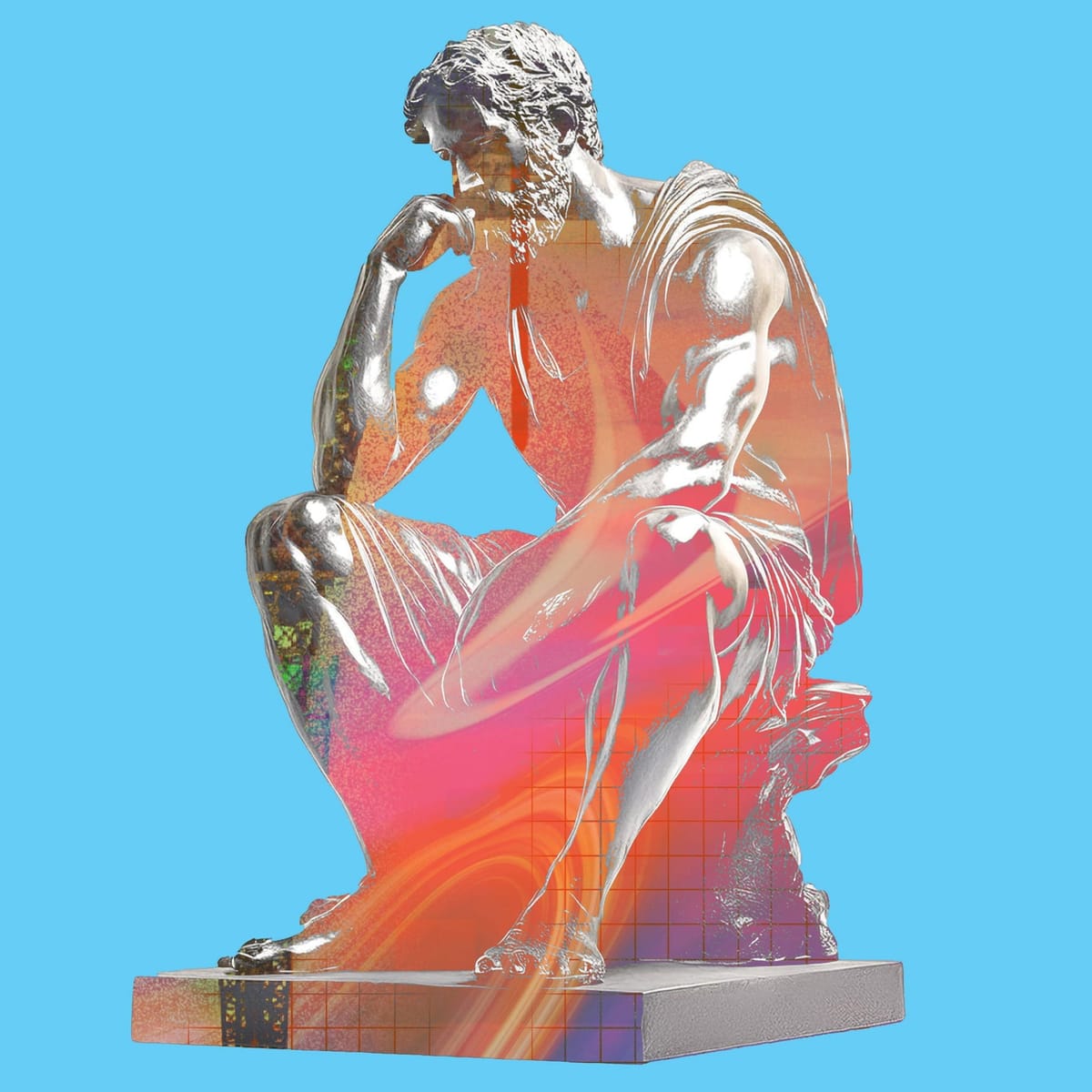
COMMENTARY By Hannah Ismael
The release of OpenAI’s GPT-4 in the spring of 2023 came with a curious disclaimer in its technical report: “[T]his report contains no further details about the architecture (including model size), hardware, training compute, dataset construction, training method, or similar.” The technical information, previously made open—hence the company’s name—was now made secret. The New York Times filed a lawsuit against the company that called out the decision, but OpenAI’s chief scientist defended it as “secrecy on commercial grounds.”
The opportunity to decide how a model gets built, especially a tool used as frequently and widely as generative AI, is a position of great power. But trade secrecy closes off that power to all but a few individuals. It also makes the tool a black box. Allowing AI experts and civil society to pry into that black box can ensure that models are more representative of diverse perspectives and help catch and address potential harms AI might pose to society.
Trade secrecy, interestingly enough, not only allows for secrecy but, through the matter of its legal requirements, encourages it. The laws around it have a fundamental requirement that “reasonable efforts” be taken to prevent the release of the trade secret. This compounds the effects of the secrecy regime.
Noncompetes and NDAs are measures frequently taken to achieve the end of reasonable efforts, but they also prevent the free movement and flow of already limited technical expertise. Moreover, it’s hard to ascertain whether a trade secret is actually worthy of that distinction without publicly disclosing it. This is, as University of Chicago legal scholars have called it, “trade secrecy’s information paradox.”
Furthermore, IP and trade secrecy can actually harm the very innovation they aim to foster by gatekeeping for a landscape with only a few players. In the case of AI, this can impact model quality down the line. For example, Stable Diffusion, the image-generation model, often produces a distorted version of the Getty watermark, presumably after being trained on watermarked photos (a practice for which it is being sued). Applications built on top of this model or companies that integrate it into their workflow are at risk of reproducing the error. The error is an example of how algorithms in a consolidated market can produce erroneous results that then become embedded and amplified downstream.
One way to address the high barriers to entry and the concentration of the market is by encouraging global investment in public infrastructure across the AI supply chain. Given the purposes AI serves for the public, it would be reasonable for individual countries to invest in publicly accessible hardware, software and data—or Public AI—for open-source organizations. This can exist as grants provided by governments to organizations seeking to democratize access to these resources (such as by supporting individuals who are creating cheaper proprietary datasets) or to individuals seeking to create an open model and needing funding to access downstream resources. The UN has already carved out funding for this purpose in its Governing AI for Humanity Report, though whether it will actually be implemented remains to be seen.
However, resolving market concentration doesn’t fix AI’s black box problem; only a systemic shift within our approach to disclosure can achieve this. European transparency legislation mandates that AI companies produce documentation describing how their models are trained, how they function and what risks they pose. This sort of partial openness forces companies to develop records of their information in a way that allows civil society an opportunity to decide whether or how to investigate harms. It also offers regulators greater clarity in understanding whether claims are valid. The act aims to balance transparency and intellectual property, allowing companies to document how their models work without truly revealing the “secret sauce.” Of course, transparency documentation has its own flaws, namely that it may produce another opportunity for firms to self-govern. However, it acts as a starting point for legislators to reconsider: Is secrecy really achieving what it set out to do?
As a global AI policy researcher at Mozilla, Hannah Ismael explores the intersection of privacy, transparency and market dynamics in AI. Previously, she served as a policy research fellow at the University of Southern California's Center for Generative AI.